NPUs disrupting Nvidia AI dominance
Tuesday, July 23, 2024
![]() |
Brittany Hainzinger |
According to DAI Magister, NPUs disrupting Nvidia AI dominance have emerged as their main rival. The semiconductor market, driven by Generative AI, is worth over $600 billion and is expected to hit $1 trillion by 2030. Nvidia leads with its high-performance GPUs, but their cost and energy use raise sustainability concerns.
The semiconductor market is projected to reach around $400 billion by 2030*, with AI semiconductors expected to constitute over 30% of this market, up from 20% today. AI has recently dominated headlines with the launch of ChatGPT, Claude, and a host of other GenAI platforms. This has prompted countless companies to examine how they can implement AI into their products, from robotics and security cameras to smart factories and electrical vehicles. However, this integration poses challenges. The processes required to implement AI algorithms overwhelm the small central processing units (CPUs) found in many devices, and the graphics processing units (GPUs) that are capable of handling them are large, expensive, and energy-intensive. This problem has given rise to a new type of chip: the neural processing unit, or NPU.
NPUs disrupting Nvidia AI dominance
NPUs are engineered to accelerate the processing of AI tasks, including deep learning and inference. They can process large volumes of data in parallel and swiftly execute complex AI algorithms using specialized on-chip memory for efficient data storage and retrieval. While GPUs are more versatile and can handle a wider range of tasks beyond AI, offering higher performance in terms of raw computing power, NPUs are domain-specific processors optimized for executing AI tasks efficiently. Consequently, NPUs can be smaller, less expensive, and more energy efficient than their GPU counterparts. Counterintuitively, NPUs can also outperform GPUs in specific AI tasks due to their specialized architecture.
Key NPU applications include enhancing efficiency and productivity in industrial IoT and automation technology, powering technologies such as infotainment systems and autonomous driving in the automotive sector, and enabling high-performance smartphone cameras, augmented reality (AR), facial and emotion recognition, and fast data processing. In data centres and machine learning/deep learning (ML/DL) environments to train AI models, NPUs are increasingly being integrated to complement GPUs, especially in scenarios requiring energy efficiency and low latency.
Despite efforts from companies like Microsoft, AWS, and Google to develop their own AI GPU and NPU chips, Nvidia remains the undisputed leader in the AI hardware market due to the superior performance and established ecosystem of their GPUs. This “race for AI” does emphasize performance, but Nvidia’s dominance in the GPU space overshadows two fundamental issues: high capex/operating costs and energy consumption related to running AI.
AI usage incurs significant economic costs
The deployment and maintenance of AI systems involve considerable financial investment. Training sophisticated AI models such as OpenAI’s GPT-4.0, requires extensive computational resources. Sam Altman himself stated that training GPT-4.0 cost over $100 million. These expenses cover the procurement of high-performance GPUs, cloud computing services, and data storage, alongside the costs of specialized human expertise in data science and engineering.
Additionally, operational costs continue to accrue post-deployment. Companies need to ensure continuous updates, maintenance, and monitoring of AI systems to keep them functional and secure. This requires hiring skilled personnel, investing in cybersecurity measures, and regularly upgrading hardware to keep up with the ever-growing data and processing demands. As a relevant reference point, Epoch AI found that the cost of the computational power required to train the most powerful AI systems has doubled every nine months.
AI drives high energy consumption
A single search query on Google, which relies on less computationally intense algorithms, uses approximately 0.3 Wh (watt-hours) of energy. In contrast, a query to an AI model like GPT-4.0 consumes significantly more energy due to the complexity and size of the model. Although precise figures for GPT-4.0 are not readily available, it is estimated that such AI queries consume up to 10 times more energy than a standard Google search. The environmental impact of AI is becoming a growing concern which is driving the development of more energy-efficient algorithms and hardware.
Initiatives are underway to mitigate the costs and carbon footprint of AI whilst remaining competitive with Nvidia’s performance, despite their market dominance and inherent capital expenditure (capex) barriers to entry.
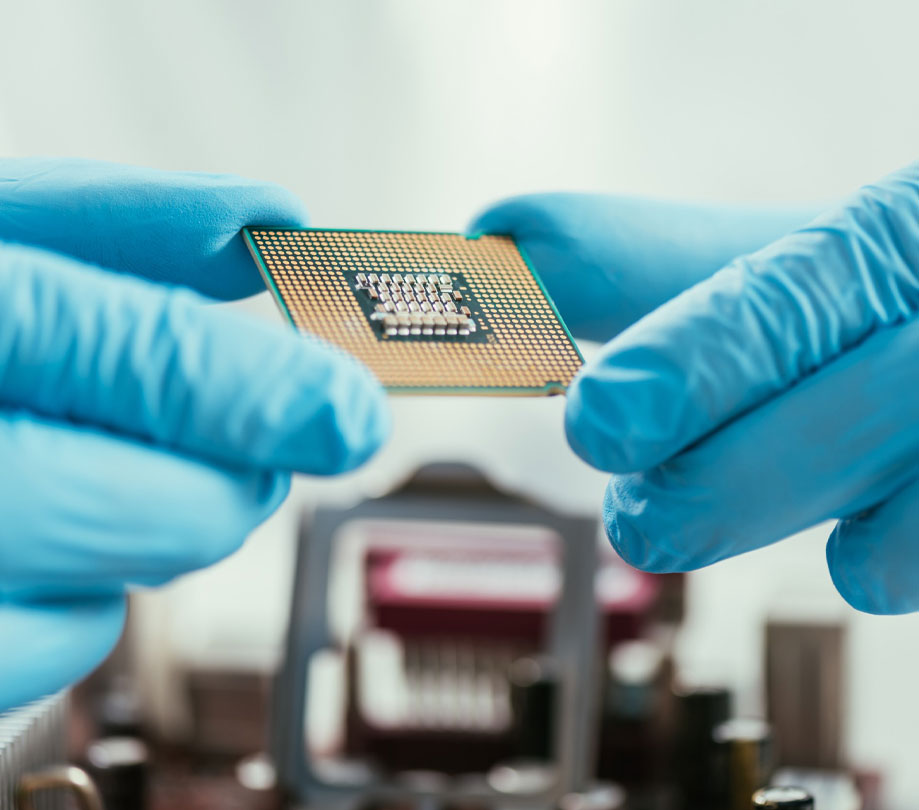
Edge inference: Cost-effective, energy-efficient alternative to server processing
The uses of AI semiconductors are bifurcated into training and inference applications. Whilst training occurs on GPUs in data centers, with Nvidia holding an astonishing 98% market share, inference can be performed on servers or edge devices. Put simply, the AI chip market can be divided into a 2×2 matrix as follows:
The AI-related NPU edge device market, estimated to reach $50 billion by 2026, is particularly noteworthy due to its emphasis on low power consumption, efficiency, and zero latency. Edge AI inference is driven by the need for enhanced data security, as reducing reliance on cloud servers minimizes the risk of data breaches. Moreover, edge devices offer real-time data processing, zero latency, and autonomy, enhancing overall performance. Cost savings are another significant factor, as minimizing dependence on costly cloud AI services can result in significant reductions in the total cost of ownership. Furthermore, reduced power consumption and carbon emissions align with environmental, social, and governance (ESG) goals.
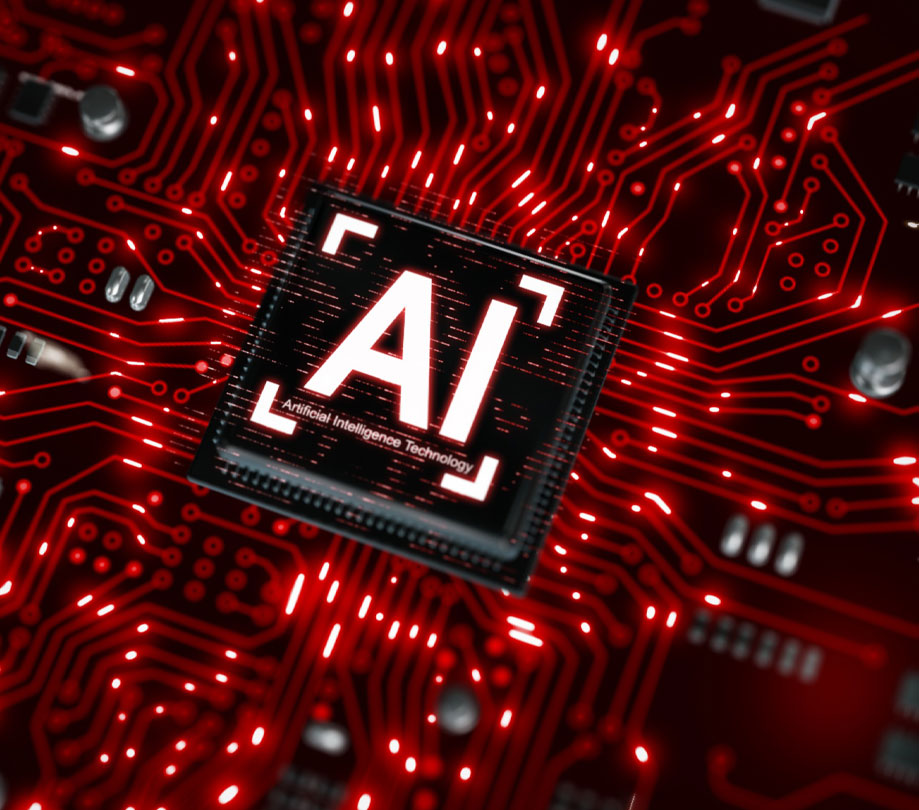
The emergence of promising companies
Start-ups from around the globe are pioneering the development of the edge AI semiconductor market by focusing on improving performance-to-power ratios, demonstrating technological innovations, and attracting investment capital to support their ambitions. These start-ups are predominantly fabless technology companies, specializing in the design and innovation of AI semiconductor solutions without owning manufacturing facilities. This business model allows them to focus finite resources on research, development, intellectual property, and their go-to-market, whilst leveraging capex-heavy foundries such as TSMC and Samsung for actual chip fabrication.
The appeal of these start-ups lies in their agility to innovate rapidly in response to market demands, unencumbered by the capital-intensive nature of semiconductor manufacturing. This flexibility not only accelerates time-to-market but also aligns with investors seeking capital-efficient, high-growth opportunities in cutting-edge technology sectors. By focusing on core competencies in chip architecture and software optimization, these fabless firms are reshaping the AI semiconductor landscape, positioning themselves as pivotal players driving the next wave of technological advancement.
In the United States, the well-developed venture capital market, particularly in Silicon Valley enables promising companies to rapidly transition from start-ups to major contenders. For instance, California-based SiMa.ai has raised an impressive $270 million to date, including a $70 million Series B led by Maverick Capital in April 2024, reaching a post-money valuation of $770 million. The company’s platform accelerates the proliferation of high-performance machine learning inference at very low power in embedded edge applications, offering push-button performance, effortless deployment, and scaling at the embedded edge. Similarly, Etched, another Californian company, secured a $120 million Series A in June 2024, led by Positive Sum and Primary Venture Partners. They provide AI-based computing hardware specialized for transformers, designed to radically cut LLM inference costs.
Texas-based Mythic has also made significant strides, raising over $175 million and achieving a post-money valuation exceeding $500 million. They offer desktop-grade graphics processing units in a chip that operates at a fraction of the cost without compromising performance or accuracy. Meanwhile, Quadric, developing edge processors for on-device AI computing, raised $31 million in its Series B round in December 2022, led by Nsitexe and MegaChips America.
Despite lacking a unified strategy for AI semiconductors, Europe has seen the emergence of promising companies. These firms are initially financed primarily by local private equity investors with finite financial capabilities, often seeking cross-border financing rounds with global investors as they scale. A notable player in this space is Axelera AI, a Netherlands-based company that announced a €63 million Series B raise in late June 2024, led by EICF, Samsung Catalyst, and Innovation Industries.
In the Middle East and Asia, Israel, and South Korea are leading the charge in AI semiconductor development. Israel, due to security concerns, has nurtured its own AI semiconductor champion in Hailo. Founded in 2017, Hailo has raised $340 million from investors including Poalim Equity and Gil Agmon, reaching an impressive post-money valuation of $1.2 billion in April 2024. The company’s AI processors are designed for running deep learning applications on edge devices, providing efficient and low-power solutions suitable for various devices, from smartphones to industrial machines.
South Korea, under its "semiconductor superpower achievement strategy," is officially supporting the emergence of future AI leaders by providing necessary funding to innovative AI companies, ensuring AI independence from Western players. DeepX, a South Korean developer of next-generation neural networks, has raised over $100 million, reaching a post-money valuation of over $500 million. Investors in its May 2024 Series C round included Timefolio, Skylake, BNW, and Aju IB. DeepX’s units are designed to maximize memory bandwidth, allowing edge devices to scale efficiently and sustainably.
OpenEdges, another South Korean company, went public in Q3 2022 and currently boasts a market capitalization of $325 million. Its success is primarily attributed to its integrated AI Edge computing IP solutions, comprised of a total memory system coupled with an AI platform IP solution combining NPU IP and Memory System IP. Mobilint, although smaller with $24 million raised to date, shows significant growth prospects offering AI-powered chip technology designed for accelerating deep neural networks-based AI algorithms and applications. It provides accelerated AI solutions through vertical integration of co-optimized algorithms, software, and hardware technologies, thereby enabling clients with performance, programmability, and end-to-end acceleration of integrated semiconductor services for autonomous driving.
This global landscape of AI semiconductor start-ups demonstrates the sector’s dynamism and potential, with each region contributing unique strengths and innovations to the rapidly evolving field.
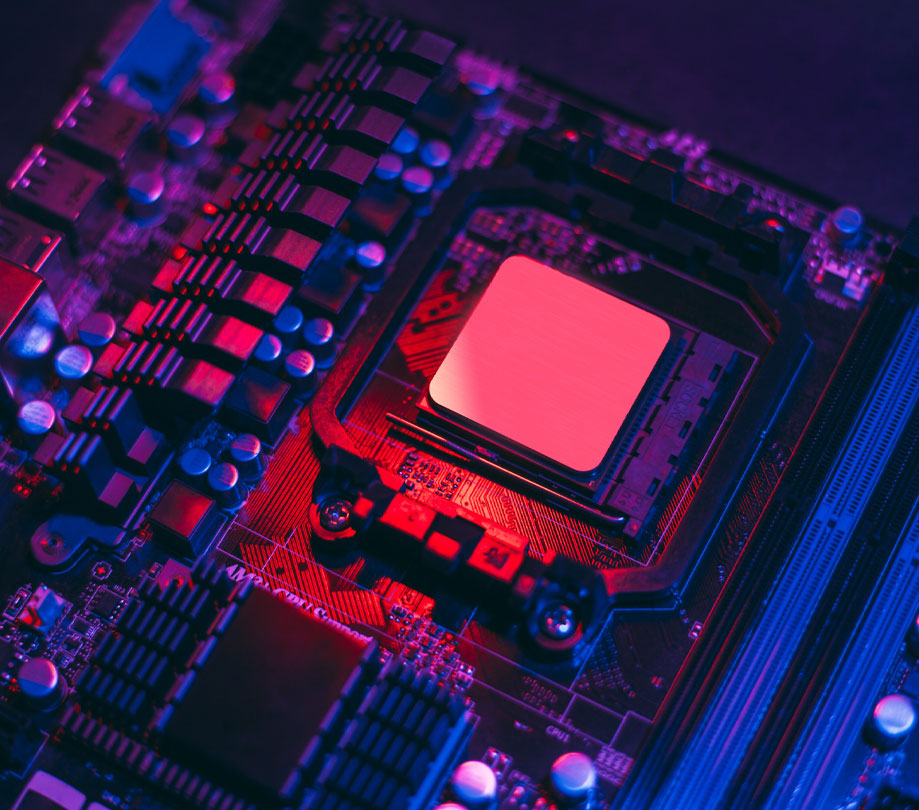
AI semiconductor fundraising to rise with cost-effective, energy-efficient edge solutions rivaling Nvidia
Nvidia’s dominance in the AI training market is unlikely to wane soon, given its superior performance and established ecosystem. Their cutting-edge GPUs and comprehensive software support make them the go-to solution for many data centers and high-performance computing applications.
Executing inference on edge devices offers a compelling alternative, addressing the pressing issues of cost, size, and energy consumption. Edge AI semiconductors provide significant benefits such as real-time processing, enhanced data security, and reduced reliance on cloud infrastructure. These factors contribute to lower total costs of ownership and minimized carbon footprints, making edge AI solutions particularly attractive for various applications, from industrial IoT to autonomous vehicles.
We expect fundraising activity in the AI-related NPU edge device sector to continue its upward trajectory. Several factors will drive this momentum: the growing importance of AI in almost all industries, increasing investments in R&D, and a surge in demand for high-performance, low-power chips. Moreover, with larger tech giants like Microsoft, AWS, and Google actively seeking to develop or acquire AI chip technologies, market consolidation is on the horizon. These tech behemoths are not only seeking to expand their capabilities but also to ensure they remain competitive against Nvidia’s formidable presence.

Become a subscriber of App Developer Magazine for just $5.99 a month and take advantage of all these perks.
MEMBERS GET ACCESS TO
- - Exclusive content from leaders in the industry
- - Q&A articles from industry leaders
- - Tips and tricks from the most successful developers weekly
- - Monthly issues, including all 90+ back-issues since 2012
- - Event discounts and early-bird signups
- - Gain insight from top achievers in the app store
- - Learn what tools to use, what SDK's to use, and more
Subscribe here