AI models are rapidly consuming power
Thursday, June 13, 2024
![]() |
Freeman Lightner |
AI models like ChatGPT require significant power, consuming over 500,000 kilowatt-hours daily for 200 million requests. AI models are expected to consume 13% of global power by 2030. Innovations like SSDs with computational storage and Arm-based computing enhance energy efficiency and performance, supporting IT sustainability.
AI and machine learning models, like ChatGPT, require a large amount of power due to the intensive processing involved. ChatGPT handles 200 million requests daily, consuming over 500,000 kilowatt-hours of electricity – 17,000 times higher than the average daily consumption of a U.S. household, of 29 kilowatt-hours.
AI models use extensive data for training, and their energy consumption rises with the complexity and popularity of the model.
- Training one AI model consumes more power than 100 households use annually.
- By 2030, AI model development will consume 13% of global power usage, contributing to 6% of global carbon emissions.
This combination of data-heavy technology and its constant need for more energy to handle complex data processing requires changes to IT architecture.
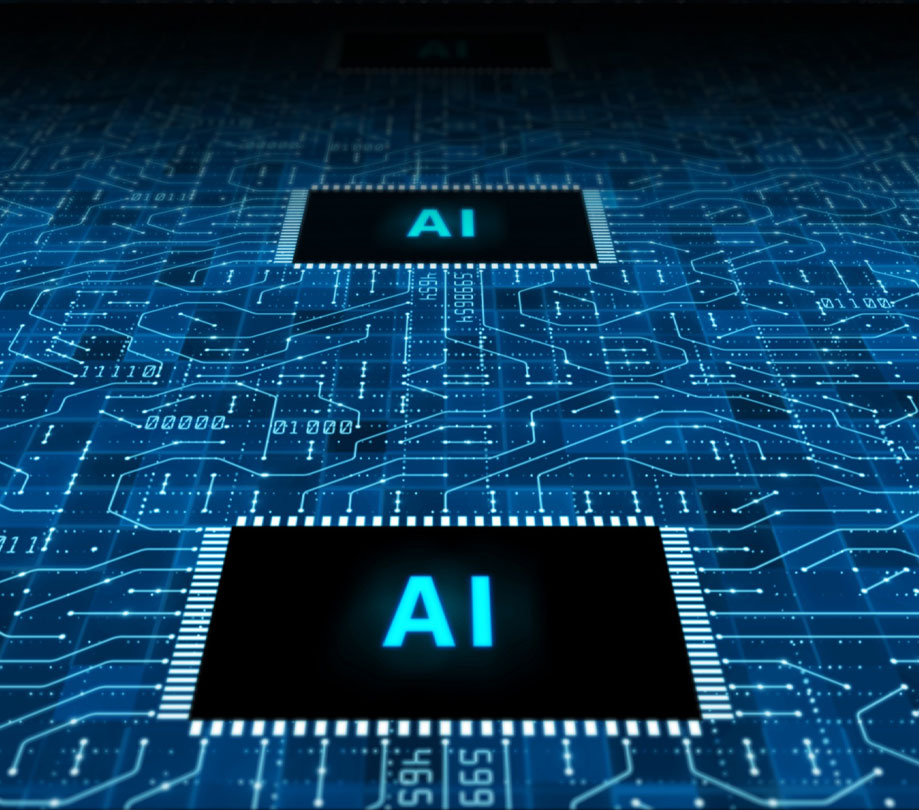
Boosting AI efficiency with computational storage and arm-based tech
Innovative solutions like SSDs with computational storage and Arm-based computing enhance performance and energy efficiency. These technologies optimize data processing and reduce energy consumption, supporting sustainability efforts in IT infrastructures.
"Managing large AI datasets presents significant storage efficiency and power challenges. Enhancing the storage, memory, and GPU pipeline with computational storage-enabled SSDs is crucial for companies to achieve energy efficiency and sustainability goals," explains JB Baker, Vice President of Products at ScaleFlux.
- Computational storage solutions like SSDs improve enterprise data centers with high-speed performance for critical decision-making, offering rapid data access, energy savings, and scalability by processing data on the drive, reducing latency, and enhancing privacy and security.
- Arm-based computing, featuring an efficient RISC microprocessor architecture with lower power consumption and scalability, makes it ideal for AI and machine learning tasks due to its superior performance and reduced power requirements compared to traditional x86 architectures.
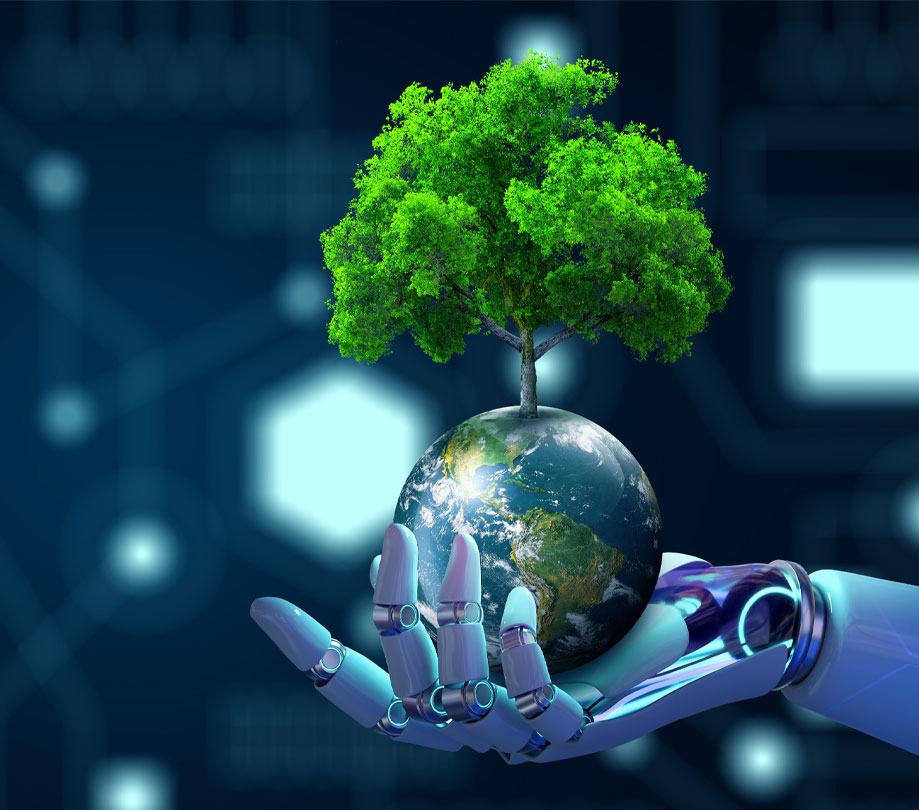
Sustainable AI: Computational storage and arm-based solutions
"Innovative and reliable technologies facilitate the seamless integration of AI acceleration into existing data infrastructures, allowing computational storage solutions to balance technological ambition with environmental stewardship," concludes Baker.

Become a subscriber of App Developer Magazine for just $5.99 a month and take advantage of all these perks.
MEMBERS GET ACCESS TO
- - Exclusive content from leaders in the industry
- - Q&A articles from industry leaders
- - Tips and tricks from the most successful developers weekly
- - Monthly issues, including all 90+ back-issues since 2012
- - Event discounts and early-bird signups
- - Gain insight from top achievers in the app store
- - Learn what tools to use, what SDK's to use, and more
Subscribe here